Before ChatGPT and other artificial intelligence (AI) large language models exploded on the scene last fall, there were AI art generators, based on many of the same technologies. Simplifying, in the context of art generation, these technologies involve a company first setting up a software-based network loosely modeled on the brain with millions of artificial “neurons.” Then, the company collects millions of data digital images, oftentimes scraped from the web. Finally, it runs the images through the neural network. The network performs billions (or even trillions) of mathematical operations on the data samples, measuring various features of the images and relationships between them, and then updating the values of the artificial neurons based on those calculations. This final step is called “model training” and it enables AI art generators to create entirely new images, typically in response to a user typing in a text prompt, as explained further below. Online digital images used as training data are an indispensable part of this process.
Andrew Neel for Pexels
OpenAI, the company behind ChatGPT, released the DALL-E text-to-image art generator in January 2021 and its successor, DALL-E 2, the following April. Two other text-to-image AI systems, Midjourney and Stable Diffusion, came out in 2022. It is well known that these models were created by training on millions of digital images downloaded from the web. This article has two goals: to provide a reader-friendly introduction to the copyright and right-of-publicity issues raised by such AI model training, and to offer practical tips about what art owners can do, currently, if they want to keep their works away from such training uses.
A GENTLE PRIMER ON GENERATIVE AI ART MODELS
Before diving in, it helps to have a big-picture sense of how generative AI art models are built and how users interact with them. The technical details are fascinating, but too complex to dwell on. Here’s the bare technological minimum to let us get to work:
- From the user’s perspective: You type in some text, e.g., “A vase of flowers with some orchids and other pretty things. Make the vase emerald blue, and the final image photorealistic.” You hit enter. The model runs, using conceptual linkages created during its training between millions of word labels and billions of image features to synthesize a new work, prompted by the criteria you typed in.
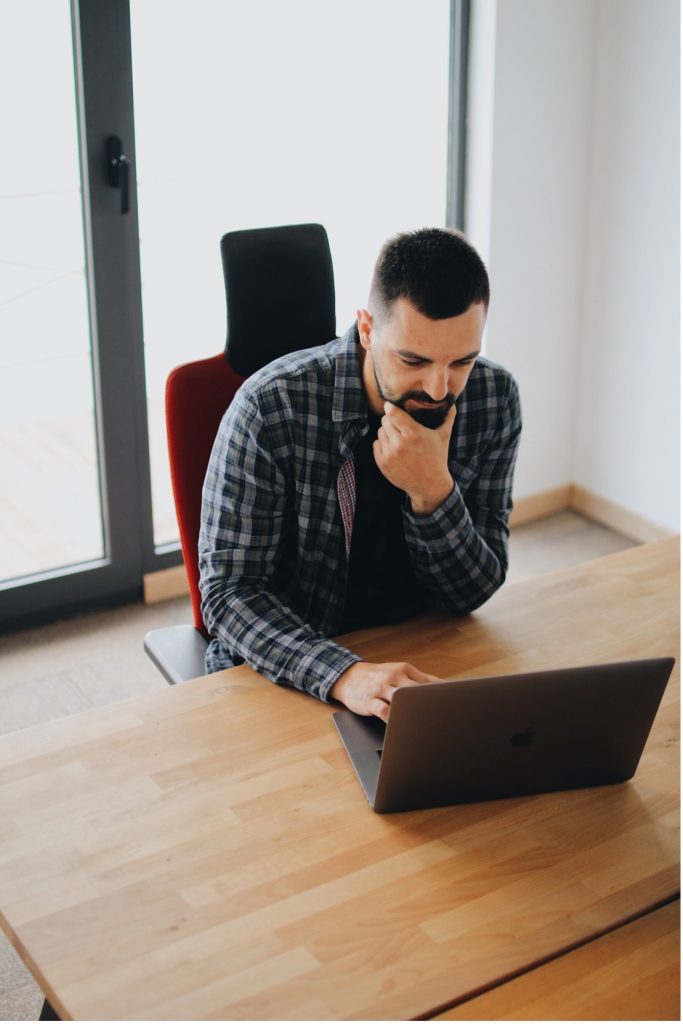
Djordje Petrovic for Pexels
- Under the hood: As noted above, AI art generators are virtual neural networks defined by billions of numbers called “weights” or “parameters.” Model developers set those parameters by running millions of images, along with text labels for each image, through training algorithms. These training images aren’t stored as perfect bit-by-bit digital replicas. They are compressed into complex mathematical entities (vectors), each of which is a huge matrix of numbers. The word labels are similarly converted (“embedded”) into vectors and combined with their corresponding image vectors. The training algorithms then run each image-word vector through a series of incremental steps, first gradually adding and then gradually removing random noise from the vector. Through this “diffusion” process, the model adjusts the numerical values of its parameters to capture the conceptual linkages between the word concepts and image features. It may seem like magic, but it is a chain of probabilistic mathematical operations run on an incomprehensively massive scale.
Because of the intentional randomness inherent in this diffusion process, only rarely will an AI model output a copy of an original training image (or something close to it) in response to a text prompt. Even in those rare cases, close reproduction typically occurs only when a user deliberately forces that result through careful prompt selection. So if outright copying is unlikely at the output stage, what are the copyright and right-of-publicity issues at stake? We’ll focus on two of them.
TRAINING ON COPYRIGHTED ARTWORK
The first big issue many in the creative and tech industries are grappling with is the permissibility of reproducing training images as an intermediate step in the training process. Many text-to-image generators are trained on massive datasets, such as LAION-5B, that include many copyrighted images. Copyright protects against unauthorized electronic reproduction. The AI model’s neural-network parameters do not store digital copies of a training image, but interim copies are typically made temporarily during training, usually in the stage of converting images to vectors. At the same time, copyright has a fair use doctrine, permitting certain copying without permission of the owner based on balancing four factors, like whether the copier’s use is transformative and whether the copying would impact the value or potential market for the image. So the question arises: When a model developer copies a digital image for training—but the model never outputs that same original image—should the intermediate-step copying be excused by fair use?
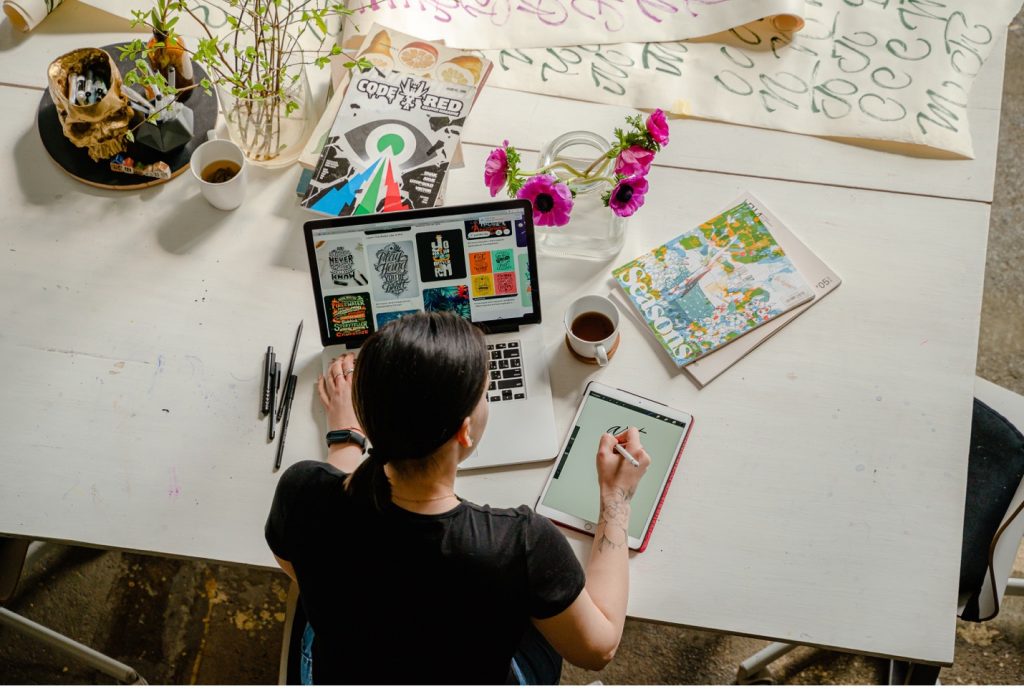
Antori Shkraba for Pexels
IMITATING ARTISTIC STYLE
The other core issue is that text-to-image AI art generators often can reproduce elements of an artist’s style, even when the content of the synthesized image is not at all similar to the original work. Think of Van Gogh’s “Starry Night” and its distinctive stylistic aspects—the swirling, strong brushstrokes; the tones of the yellows and blues; and the soft light emanating seemingly from underneath. Now take that stylistic “skin” and apply it to a wholly different scene, a bowl of fruit, or an airport runway. Van Gogh’s style may be carried over, but a copyright claim is challenging. Because the content of the picture is fundamentally different, the work is not substantially similar. Generally, copyright protection does not extend to abstractions like styles standing alone.
Style transfer in AI art generators has received considerable attention. The best-known example is digital artist Greg Rutkowski, recognized for his dark moody fantasy scenes used in games like Dungeons & Dragons. At one point last fall, users had explicitly prompted Stable Diffusion to create images “in the style of Greg Rutkowski” over 93,000 times. Because the content of the synthesized images was different, a copyright claim would be a stretch. Another possibility is a right-to-publicity claim. Generally, this right guards against an unauthorized commercial use of a person’s name, likeness, persona, or identity. But courts, at least so far, have not clearly decided whether a visual artist’s style can qualify as a kind of “persona” or “identity,” nor have they discussed what level of distinctiveness may be necessary to create such “personas” or “identities,” nor how such distinctiveness is to be measured.
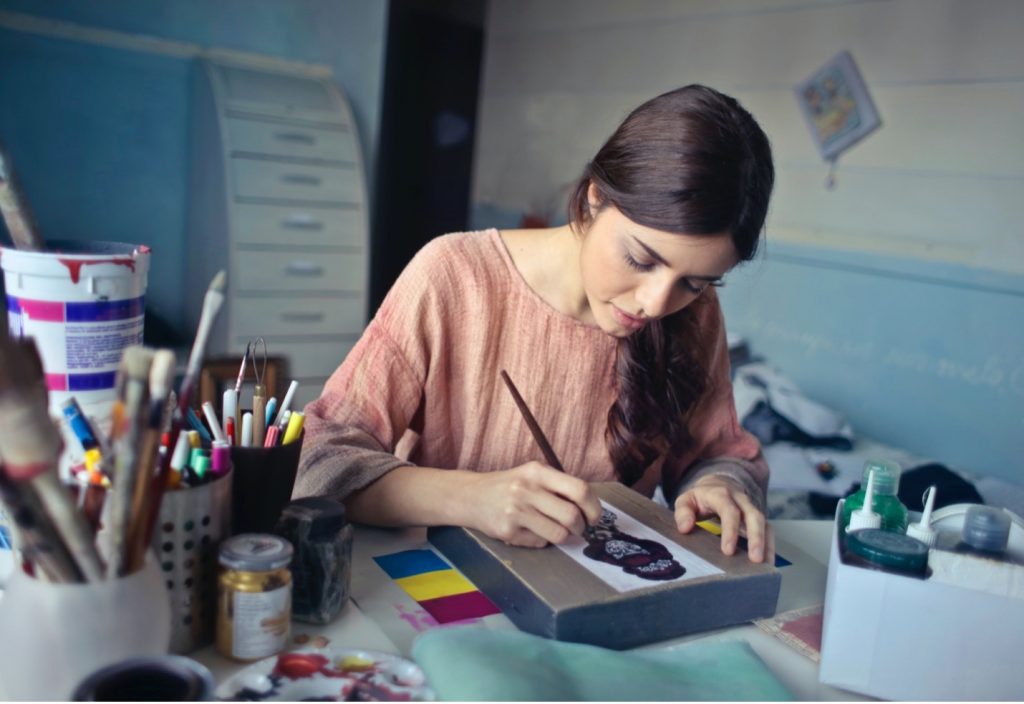
Andrea Piacquadio for Pexels
PRACTICAL TIPS
Eventually, courts will weigh in on these training data and style transfer issues. Earlier this year, Getty Images sued Stability, the company behind the Stable Diffusion model, in Delaware federal court over the use of Getty copyrighted photos in Stable Diffusion’s training. Similarly, a group of visual artists sued both Stability and Midjourney in California federal court over their copyrighted artwork and included right-to-publicity claims. But those cases are still in their early stages. It will take time before we receive clear, actionable guidance from the courts. In the meantime, what can artists concerned about style imitation nor training usage do?
Your current options are mostly technological, not entirely satisfactory, but better than nothing. First is detection. Software tools exist to help you determine whether an AI art model used a training dataset that included your image. The startup Spawning offers the free site Have I Been Trained? where you can upload your file and check to see whether it exists in the LAION-5B dataset used to train Stable Diffusion and other AI art generators. Or, if your artwork is highly distinctive, you can try to “hack” the model by experimenting with different text prompts to induce the model to output something close to your original image.
The next step is communication. If you believe your artwork was improperly used to train an AI model, you can write the developer’s legal department, identifying your images with specificity and asking them to remove those images from the training dataset. There’s no guarantee your request will be honored, but you will have provided notice of your objection. There are also ways to communicate your anti-scraping intent through metadata instructions. If you publish your artwork on your website, make sure the site’s “robot.txt” file includes tags prohibiting web crawlers. OpenAI recently announced that its GPTBot scraper would respect these tags. Similarly, DeviantArt, an online site where artists showcase and sell their digital works, includes a “NoAI” HTML tag by default for uploads to its platform. Also for individual digital images, the Adobe-led Content Authenticity Initiative has issued a technical standard—C2PA “content credentials”—for cryptographically binding a metadata “manifest” to images. The newest version of the standard (1.3) allows users to include an instruction in the manifest prohibiting AI model training on the image. Of course, these metadata systems are not ideal solutions, because they are not legally mandated or widely adopted yet. But they offer a promising path for future protection.
A final step you can take is prevention. Academics are developing and have released tools that would allow artists to electronically modify their digital artwork and photo files to inhibit their downstream reproduction. To specifically address the issue of style mimicry, a team at the University of Chicago developed Glaze, a software program that manipulates individual pixels to alter the style. The change is imperceptible to the human eye, but the AI art model is “tricked” into thinking the image has a different style, cubist instead of photorealistic, for example. Glaze is now publicly available to artists through the University of Chicago website. A similar tool, still in the prototype stage, is PhotoGuard from the Massachusetts Institute of Technology. PhotoGuard alters digital photos, also at the pixel level, so that any AI-synthesized outputs using those photos have degraded appearances—key areas of the output may be grayed out, for instance.
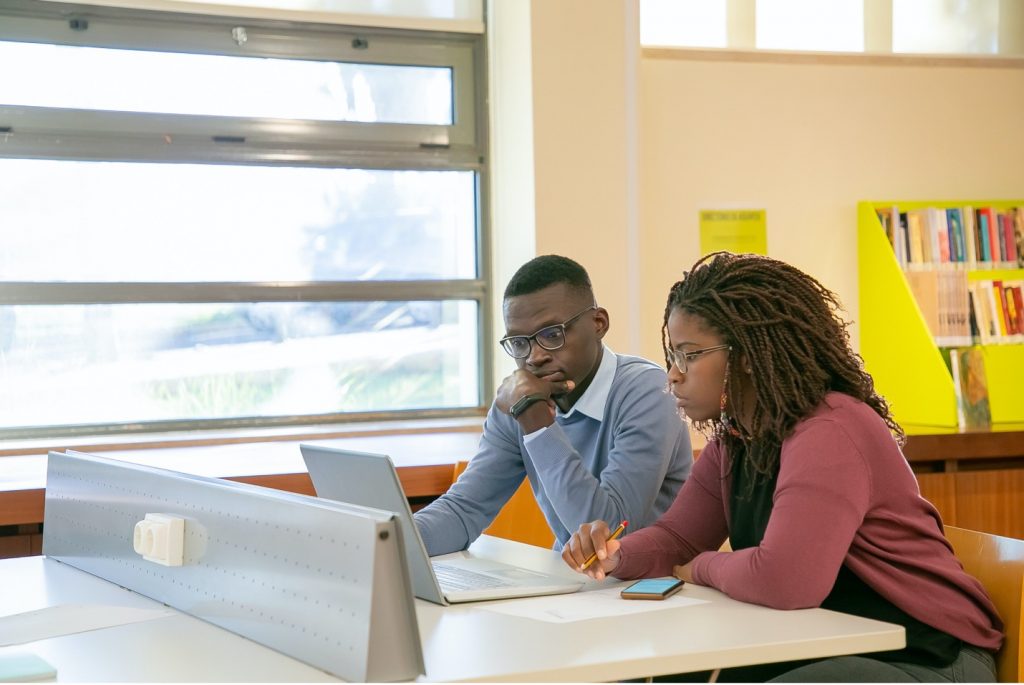
Campus Production for Pexels
PARTING THOUGHTS
Issues around artistic style transfer and the use of copyrighted works as AI training data will be with us for the foreseeable future. Legitimate, provocative, vital debate between creators, developers, and the public continues in the news, in legislatures, and in the courts. But while we wait for the right balances to be struck, it behooves visual artists to understand both the basics of how AI image generators work and the technological tools available to them to help control unauthorized uses of their works. The times are ever-changing―and we must keep up with the times.
Author Bio
Aleksander J. Goranin is a partner in the intellectual property practice of Duane Morris LLP. He is a software copyright and patent litigator and counselor, specializing in technology-driven cases, high-stakes problems, and turning the complex into the understandable. Alex is active in the leadership of the Copyright Society and co-chairs its AI Series of educational programming. At Duane Morris, he helps lead the firm’s AI Steering Committee and publishes its biweekly newsletter summarizing legal developments in artificial intelligence, The AI Update.